"Anything that can think or act like human"
1936
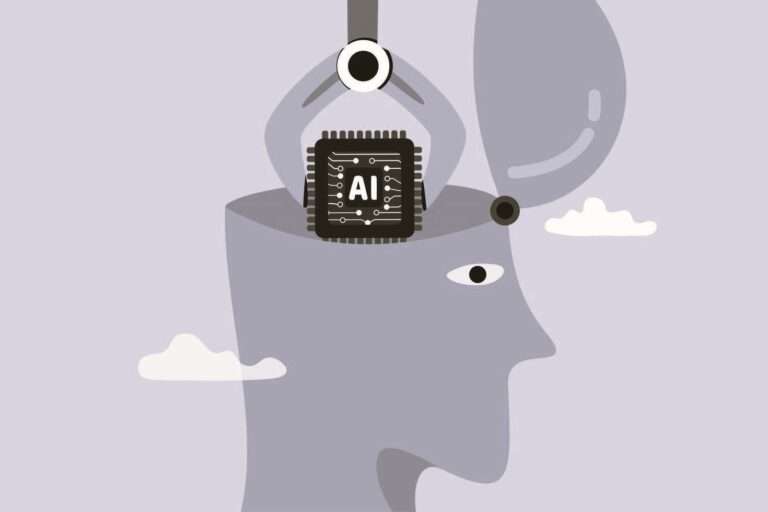
People were fascinated with the idea of building machines that could think and act like humans. The idea of machine intelligence sparked in the human brain long before the actual digital computers were invented. In 1936, Alan Turing first gave the idea of a thinking hypothetical machine, called the Turing machine. This concept basically showed the way to embed intelligence into a machine through a set of defined rules.
1950
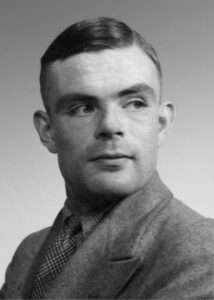
1955
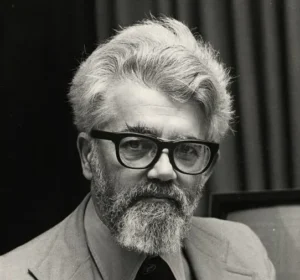
John McCarthy, known as the Father of AI, coined the term Artificial Intelligence (AI). He defined AI as the science and engineering of making intelligent machines. His work was mainly focused on understanding human consciousness and self-awareness of the choices that we have. He tried to mimic the same in AI as much as possible and produced interesting work on robot consciousness and free will.
1958
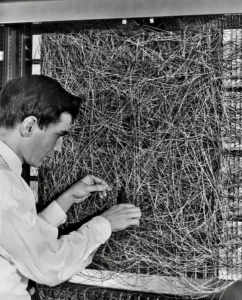
1974
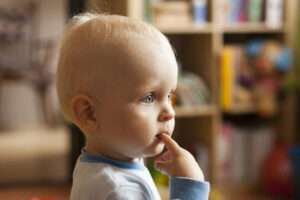
Soon, machines were able to solve logical problems like playing checkers or solving algebra, which was considered ‘hard’ for a normal human being to solve. Leading researchers assumed this was a sign of intelligence that can easily imitate humans. But they were wrong about it. The ‘hard’ logical problems were actually easier to solve as compared to the ‘easy’ problems of imitating the skills of a one-year-old baby, in terms of mobility and perception. This is interestingly coined under the term “Moravec’s paradox“. Without realizing the complexity involved in basic human tasks like walking or picking a pencil, people hyped the potential of AI in imitating human behavior. As they realized the true nature of problems, this inevitably lead to the first downfall of AI (1974-1980), which collapsed its value in the perspective of government bureaucrats. This pushed down the research funding in AI and is termed the first AI winter of the 20th century.
1987
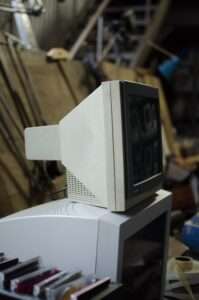
2000
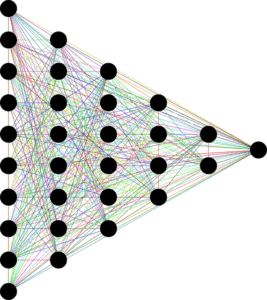
2015
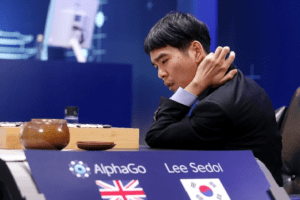
2021
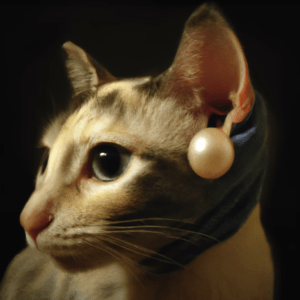
2 Responses
I have been browsing online more than three hours today yet I never found any interesting article like yours It is pretty worth enough for me In my view if all website owners and bloggers made good content as you did the internet will be a lot more useful than ever before
Thank you!